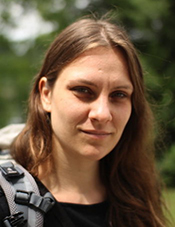
Abstract: High-dimensional, nonlinear, multi-scale phenomena, such as turbulent fluid flows or the spread of infectious diseases, are ubiquitous; however, we still lack a good understanding of these as analytically tractable models remain an exception. The lack of simple equations and an unprecedented amount of available high-fidelity data are leading to a paradigm shift in how we interact with complex nonlinear systems. Leading approaches stem from data-driven methods which have the potential to discover new mechanisms, models and control laws and are driven by the tremendous advances in computing power, sensor systems, and advanced algorithms in machine learning.
In this talk, I will discuss recent advances in data-driven architectures for system identification and control leveraging sparsity-promoting techniques and machine learning. The first part is related to the emerging field of linear operators in dynamical systems, such as the Perron-Frobenius and Koopman operators. These seek to embed nonlinear dynamics in a globally linear representation, providing a compelling mathematical framework for the linear estimation, prediction, and control of strongly nonlinear systems. I will demonstrate how we can extract physical information and incorporate control in these representations and discuss some of the challenges and successes in the context of high-dimensional fluid flows. The second part is focused on how we can infer interpretable and generalizable models for control directly from data using library expansions and sparse regression. The resulting SINDYc models have the ability to enhance the performance of model predictive control (MPC), based on limited, noisy data; thus, making it viable for online training and execution in response to rapid system changes.
Biosketch: Eurika Kaiser is a Moore/Sloan & WRF Innovation in Data Science Postdoctoral Fellow at the eScience Institute and the Mechanical Engineering Department at the University of Washington. Her research focuses on advancing theory and methods for the modeling and control of nonlinear systems using data-driven and machine learning methods, with a focus on fluid dynamics. She received her Diploma degree (M.Sc.) in Physical Engineering at the Technical University Berlin, and her Ph.D. at the University of Poitiers (France).
Date/Time:
Date(s) - Feb 27, 2020
2:00 pm - 3:00 pm
Location:
38-138 Engineering IV
420 Westwood Plaza Los Angeles CA 90095